Top 20 Must-Read Software Trends Reports for 2023
Life in First Principles
Time
Time is the one variable that nobody has any control over. There are 24 hours in a day, 365 days in a year. Time offers the same equal consistency to everybody, but at different lengths. We are born, we live, and we die. All you can do is maximise the other variables in the time given to you, in a way meaningful to you.Energy
Being alive is a prerequisite, but not sufficient to reach happiness. Given a lifetime, to maximise your purpose and happiness, depends on your energy levels. Energy is the ability to do things, physically or mentally. Having a body sufficiently healthy that will enable you to pursue your purpose. For some, this can be a physically strong body, having a good sleep, healthy diet, and regular exercise. For others, it can be sufficient to be able to get up from the bed and hold a pen. And for some even the ability to express your thoughts through a computer device (such as Stephen Hawking). Energy levels vary from person to person, but so are the energy needs. Energy is not a "have or have not" constant like life is, it is rather a variable that tends that changes every moment, and tends to go down with age. Energy is the multiplier that lets you get the best out of the time given to you.Focus
Being lucky to be alive, and having sufficient energy, gives the optionality to spend your attention in many ways. Focus is about how we use our time and energy in a directed way. It is the ability to concentrate our attention in a direction that makes us happy, or spread and waste in the universe in a way benefiting others or nobody.Focus is the variable that we have most control over, and the variable that has the biggest power to change our life. Used in a purposeful way, even in short lifespan and limited energy levels, it has led to personal fulfilments, or human achievements that are remembered throughout millennials. Used purposelessly, can lead to wasted long life full of energy, and many regrets in a death bed.
The happiness formula
If time is a yes/no constant, and the energy level is a multiplier for every moment, then focus is the exponent of all. Every moment, we are alive, we have a certain energy level that we can use for something purposeful or waste for nothing. Then we have recharge again. Every moment we have the ability to focus our energy to things that matter to us, or waste it aimlessly. Life happiness is the sum of all moments we had, with sufficient energy to help us focus on things that makes us happy.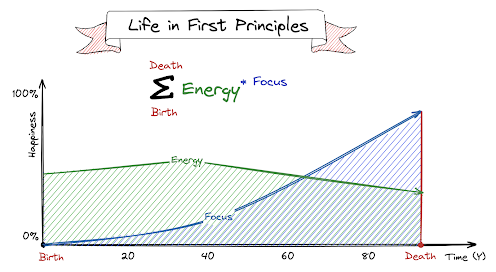
we focus our energy towards our purpose
How does Knative project compare to Dapr?
How does Knative project compare to Dapr?
Both Dapr and Knative projects help create and run cloud-native applications on Kubernetes, but differ in important aspects. I thought I'd quickly share where these projects overlap and complement each other from a user point of view. If you prefer, you can read this post as a twitter thread too.Knative extends Kubernetes with serverless containers (scaling to and from 0) and helps you connect applications declaratively. Dapr helps developers implement reliable connected distributed applications quickly.
Community
Knative originated from Google, whereas Dapr from Microsoft. Today both projects are incubating at CNCF. Both projects have growing communities and are within top 20 active CNCF projects (Dapr #10 and Knative #17)Primary focus area
➤Knative extends Kubernetes with serverless containers by taking care of runtine networking (sync/async), autoscaling (to/from zero), and app revision tracking.Target user
➤Knative serving can be used by Ops to auto-scale and release apps (with traffic splitting). Knative eventing and functions can be used by devs to build, deploy apps, and connect external systems and event-driven containers.Supported platforms
➤Knative runs only on top of Kubernetes and a network layer such as Kourier, Istio, Contour.Deployment model
Developer experience
Operational experience
Top features
Feature overlap
Sweet spot
Summary
Those are the key differences between Dapr and Knative I'm aware of. If you know other differences, share those on the twitter thread. We @diagridio are actively working on both projects and exploring how they complement each other and integrate with the greater CNCF ecosystem.Apache Kafka Landscape
- Active Apache Kafka projects list: https://bit.ly/kafkaland
- Self-service form to add a project: https://bit.ly/kafkaland-form
Apache Kafka landscape projects
The projects are organized into main categories of the Kafka ecosystem, including Kafka distributions, stream processors, client libraries, connectors, schema registry, replicator, rest proxy, and management tools. Each project includes information on its license, programming language, and deployment types. Additionally, statistics such as GitHub stars or downloads are provided too, but it should be noted that these might have become outdated. A simple report generated from the list is available for reference.
Apache Kafka project stats
Discovering other active projects within the Apache Kafka ecosystem can be time-consuming. However, the data provided here is available for you to explore and use as you desire, saving you time and effort. If you wish to add any additional projects, tools, or services related to Apache Kafka, use the provided self-service form.
If you found this content useful, share and help spread this list to others in the Kafka universe. I hope to see you at Kafka Summit London this year. If my talk is accepted 🤞, I will be discussing the topic of creating "Polyglot Microservices with Dapr and Kafka" in more detail.
Unbundling: The Natural Evolution of Tech Stacks
![]() |
The unbundling of the tech stack |
- Microservices emerged as a response to the limitations of monolithic architecture, which can be inflexible and difficult to scale and maintain as an application grows. By breaking a monolithic application into smaller, independent services, it becomes possible to develop, deploy, and scale each part of the application independently, leading to faster development cycles and greater flexibility.
- Hexagonal architecture was developed to improve the flexibility and maintainability of 3-tier applications by decoupling components and providing standardized interfaces for interacting with them.
- Domain-driven design (DDD) is a software development approach that can help break down a monolithic application into smaller, loosely-coupled modules that represent different business domains or contexts.
- Micro frontends architecture is a design approach in which a large, monolithic frontend application is broken down into smaller, independent modules that can be developed and deployed separately.
- JAMstack decouples the frontend and backend of an application by separating the HTML, CSS, and JavaScript that make up the user interface from the server-side code and databases that power the application, allowing easier maintenance as changes to one part of the system do not necessarily require changes to the other.
- A service mesh decouples the networking responsibilities of a distributed application, such as routing, load balancing, and service discovery, from the application itself, allowing developers to focus on building business logic and features without worrying about the underlying network infrastructure.
- Similar to microservices, data mesh involves decomposing a large, complex system into smaller, independent components. It decomposes data governance and management practices into smaller, independent components that can be implemented and enforced consistently across different data sources and systems.
- The 2 pizza team model is a strategy for organizing teams and work in organizations that advocates for smaller teams able to respond to changes more quickly, have better communication and collaboration, can make faster decisions and solve problems more efficiently.
First Steps with Dapr
I recently left Red Hat to join Diagrid and work on the Dapr project. I spoke about Dapr when it was initially announced by Microsoft, but hadn’t looked into it since it joined CNCF. Two years later, during my onboarding into the new role, I spent some time looking into it and here are the steps I took in the journey and my impressions so far.
What is Dapr?
- Similar to Camel, Dapr has connectors (called bindings) that let you connect to various external systems.
- Similar to HashiCorp Consul, Dapr offers services discovery which can be backed by Consul.
- Similar to Spring Integration, Spring Cloud, (remember Netflix Hystrix?) and many other frameworks, Dapr has error handling capabilities with retries, timeouts, circuit breakers which are called resiliency policies.
- Similar to Spring Data KeyValue, Dapr offers Key/Value-based state abstractions.
- Similar to Kafka, Dapr offers pub/sub-based service interactions.
- Similar to ActiveMQ clients, Dapr offers DLQs, but these are not specific to a messaging technology, which means they can be used even with things such as AWS SQS or Redis for example.
- Similar to Spring Cloud Config, Dapr offers configuration and secret management
- Similar to Zookeeper or Redis clients, Dapr offers distributed locks
- Similar to a Service Mesh, Dapr offers mTLS and additional security between your application and the sidecar.
- Similar to Envoy, Dapr offers enhanced observability through automatic metrics, tracing and log collection.
You could say, Dapr is a collection of stable APIs exposed through a sidecar and swappable implementations running somewhere else. It is the cloudnative incarnation of integration technologies that makes integration capabilities previously available only in a few languages, available to everybody, and portable everywhere: Kubernetes, on-premise, or literally on the International Space Station (I mean the edge).
Getting started
The project is surprisingly easy to get up and running regardless of your developer background and language of choice. I was able to follow the getting started guides and run various quickstarts in no time on my MacOS. Here are roughly the steps I followed.
Install Dapr CLI
Dapr CLI is the main tool for performing Dapr-related tasks such as running an application with Dapr, seeing the logs, running Dapr dashboard, or deploying all to Kubernetes.
brew install dapr/tap/dapr-cli
With the CLI installed, we have a few different options for installing and running Dapr. I’ll start from the least demanding and flexible option and progress from there.
Option 1: Install Dapr without Docker
This is the lightest but not the most useful way to run Dapr.
dapr init --slim
In this slim mode only daprd and placement binaries are installed on the machine which is sufficient for running Dapr sidecars locally.
Run a Dapr sidecar
The following command will start a Dapr sidecar called no-app listening on HTTP port 3500 and a random gRPC port.
dapr run --app-id no-app --dapr-http-port 3500
Congratulations, you have your first Dapr sidecar running. You can see the sidecar instance through this command:
dapr list
or query its health status:
curl -i http://localhost:3500/v1.0/healthz
Dapr sidecars are supposed to run next to an application and not on their own. Let’s stop this instance and run it with an application.
dapr stop --app-id no-app
For this demonstration we will use a simple NodeJS application:
git clone https://github.com/dapr/samples.git
cd samples/hello-dapr-slim
npm install
This is a Hello World the Dapr way and here is the gist of it:
app.post('/neworder', bodyParser.json(), (req, res) => {
const data = req.body.data;
const orderId = data.orderId;
res.status(200).send("Got a new order! Order ID: " + orderId); });
The application has one /neworder endpoint listening on port 3000. We can run this application and the sidecar with the following command:
dapr run --app-id nodeapp --app-port 3000 --dapr-http-port 3500 node app.js
The command starts the NodeJS application on port 3000 and Dapr HTTP endpoint on 3500. Once you see in the logs that the app has started successfully, we can poke it. But rather than hitting the /neworder endpoint directly on port 3000, we will instead interact with the application through the sidecar. We do that using Dapr CLI like this:
dapr invoke --verb POST --app-id nodeapp --method neworder --data '{"data": { "orderId": "41" } }'
And see the response from the app. If you noticed, the CLI only needs the app-id (instead of host and port) to locate where the service is running. The CLI is just a handy way to interact with your service. It that seems like too much magic, we can use bare-bones curl command too:
curl -XPOST -d @sample.json -H "Content-Type:application/json" http://localhost:3500/v1.0/invoke/nodeapp/method/neworder
This command uses the service Dapr’s invocation API to synchronously interact with the application. Here is a visual representation of what just happened:
Now, with Dapr on the request path, we get the Daprized service invocation benefits such as resiliency policies such as retries, timeouts, circuit breakers, concurrency control; observability enhancements such as: metrics, tracing, logs; security enhancements such as mTLS, allow lists, etc. At this point, you can try out metadata, metrics endpoints, play with the configuration options, or see your single microservice in Dapr dashboard.
dapr dashboard
The slim mode we are running on is good for the Hello World scenario, but not the best setup for local development purposes as it lacks state store, pub/sub, metric server, etc. Let’s stop the nodeapp using the command from earlier (or CTL +C), and remove the slim Dapr binary:
dapr uninstall
One thing to keep in mind is that this command will not remove the default configuration and component specification files usually located in: ~/.dapr folder. We didn’t create any files in the steps so far, but if you follow other tutorials and change those files, they will remain and get applied with every dapr run command in the future (unless overridden). This caused me some confusion, keep it in mind.
Option 2: Install Dapr with Docker
This is the preferred way for running Dapr locally for development purposes but it requires Docker. Let’s set it up:
dapr init
The command will download and run 3 containers
- Dapr placement container used with actors(I wish this was an optional feature)
- Zipkin for collecting tracing information from our sidecars
- And a single node Redis container used for state store, pub/sub, distributed-lock implementations.
docker ps
Run the Quickstarts
My next step from here was to try out the quickstarts that demonstrate the building blocks for service invocation, pub/sub, state store, bindings, etc. The awesome thing about these quickstarts is that they demonstrate the same example in multiple ways:- With Dapr SDK and w/o any dependency to Dapr SDK i.e. using HTTP only.
- In multiple languages: Java, Javascript, .Net, Go, Python, etc.
Option 3: Install Dapr on Kubernetes
If you have come this far, you should have a good high-level understanding of what Dapr can do for you. The next step would be to deploy Dapr on Kubernetes where most of the Dapr functionalities are available and closest to a production deployment. For this purpose, I used minikube locally with default settings and no custom tuning.
dapr init --kubernetes --wait
- dapr-operator: manages all components for state store, pub/sub, configuration, etc
- dapr-sidecar-injector: injects dapr sidecars into annotated deployment pods
- dapr-placement: required with actors only.
- dapr-sentry: manages mTLS between services and acts as a certificate authority.
- dapr-dashboard: a simple webapp to explore what is running within a Dapr cluster
Injecting a sidecar
From here on, adding a Dapr sidecar to an application (this would be Dapr dataplane) is as easy as adding the following annotations to your Kubernetes Deployments:
annotations:
dapr.io/enabled: "true"
dapr.io/app-id: "nodeapp"
dapr.io/app-port: "3000"
The dapr-sidecar-injector service watches for new Pods with the dapr.io/enabled annotation and injects a container with the daprd process within the pod. It also adds DAPR_HTTP_PORT and DAPR_GRPC_PORT environment variables to your container so that it can easily communicate with Dapr without hard-coding Dapr port values.
To deploy a complete application on Kubernetes I suggest this step-by-step example. It has a provider and consumer services and it worked the first time for me.
Transparent vs explicit proxy
Summary
Liked more
- I love the fact that Dapr is one of the few CNCF projects targeting developers creating applications, and not only operations team who are running these applications. We need more cloudnative tools for implementing applications.
- I love the non-intrusive nature of Dapr where capabilities are exposed over clear APIs and not through some black magic. I prefer transparent actions for instrumentation, observability, and general application insight, but not for altering application behavior.
- I loved the polyglot nature of Dapr offering its capabilities to all programming languages and runtimes. This is what attracted me to Kubernetes and cloudnative in the first place.
- I loved how easy it is to get started with Dapr and the many permutations of each quickstart. There is something for everyone regardless of where you are coming from into Dapr.
- I’m excited about WASM modules and remote components features coming into Dapr. These will open new surface areas for more contributions and integrations.
Liked less
- I haven’t used actors before and it feels odd to have a specific programming model included in a generic distributed systems toolkit. Luckily you don’t have to use it if you don’t want to.
- The documentation is organized, but too sparse into multiple short pages. Learning a topic will require navigating a lot of pages multiple times, and it is still hard to find what you are looking for.
Kafka Distributions Landscape
One aspect of Apache Kafka that makes it superior to other event streaming projects is not its technical features and performance characteristics, but the ecosystem that surrounds it. The number of books, courses, conference talks, Kafka service providers, consultancies, independent professionals, third-party tools and developer libraries that make up the Kafka landscape is unmatched by competing projects.
While this makes Kafka a de facto standard for event streaming and provides assurance that it will be around for a long time to come, at the same time, Kafka alone is just a cog in the wheel and does not solve business problems on its own. This raises the question of which Kafka distributions are best suited to our use cases and which ecosystem will enable the highest productivity for our development teams and organizational constraints. In this post, we will try to navigate the growing ecosystem of Kafka distributions and give you some thoughts on where the industry is heading.
Kafka for Local Development
If you are new to Kafka, you may assume that all you need is a Kafka cluster, and you are done. While this statement might be correct for organizations with a low level of Kafka adoption where Kafka is a generic messaging infrastructure, it does not represent the picture in the organizations with a higher level of event-streaming adoption where Kafka is used heavily by multiple teams in multiple sophisticated scenarios. The latter group needs developer productivity tools that offer rapid feedback during development of event-driven applications, high levels of automation, and repeatability in lower environments, and depending on the business priorities a variety of hybrid deployment mechanisms from edge to multiple clouds in production.
The very first thing a developer team working heavily with stream processing applications would want is being able to start a short-lived Kafka quickly on their laptop. That is true regardless if you practice test-driven development and mock all external dependencies, or a rapid prototyping technique. As a developer, I want to quickly validate that my application is wiring up and functioning properly with an in-memory database or messaging system. Then I want to be able to do repeatable integration testing with a real Kafka broker. Having this rapid feedback cycle enables developers to iterate and deliver features faster and adapt to changing requirements. The good news is that there are a few projects addressing this need. The ones that I’m most familiar with are Quarkus extension for Kafka and EmbeddedKafka from Spring in the Java ecosystem. The easiest way to unit test Kafka applications is with smallrye-messaging that replaces the channel implementation with in-memory connections. This has nothing to do with Kafka, but shows how using the right streaming abstraction libraries can help you unit test applications rapidly. Another option is to start an in-memory Kafka cluster in the same process as the test resource through EmbeddedKafkaCluster to use that for a quick integration test. If you want to start a real Kafka broker as a separate process as part of the resource, Quarkus can do that through Dev Services for Kafka. With this mechanism, Quarkus will start a Kafka cluster in less than a second using containers. This mechanism can validate Kafka-specific aspects of your application and ensure it is working as expected on the local machine. The cool thing about Dev Services is that it can also start a schema registry (such as Apicurio), relational databases, caches, and many other 3rd party service dependencies. Once you are done with the “inner-loop” development step, you want to commit your application to a source control system and run some integration tests on the central build system. You can use Test Containers to start a Kafka broker from a Java DSL (or librdkafka mock for C), and allow you to pick specific Kafka distributions and versions. If your application passes all the gates, it is ready for deployment into a shared environment with other services where a continuously running Kafka cluster is needed.
In this post, we are focusing only on the Kafka broker distributions and not the complete Kafka ecosystem of tools and additional components. There are other monitoring and management tools, and services that help developers and operations teams with their daily activities which we leave for another post.
Self-managed Kafka
Since our application has not reached production or a performance testing environment that requires production-like characteristics, all we want is to have a Kafka installation that is reliable enough for various teams to integrate and run some tests without involving a lot of effort to manage. Another characteristic of such an environment is to be low cost without the cost overhead of data replication and multi-AZ deployment. Many organizations have Kubernetes environments where each development team has their isolated namespace and shared namespaces for CI/CD purposes with all the shared dependencies deployed. Strimzi project - origicnally created by Red Hat has everything needed to automate and operate a Kafka cluster on Kubernetes for development and production purposes. The advantage of using Strimzi for the lower environments is that it can be managed through a declarative Kubernetes API which is used by developers to manage the applications they develop and other 3’rd party dependencies. This allows developers to use the same Kubernetes infrastructure to quickly create a Kafka cluster for individual or team uses, a temporary project cluster, or a longer living shared cluster, repeatedly through automation pipelines and processes w/o going to depend on other teams for approval and provisioning of new services.
List of Apache Kafka distributions and use cases
Self-managed Kafka clusters are not used only for development purposes, but for production too. Once you get closer to a production environment, the characteristics required from the Kafka deployment change drastically. You want to be able to provision production-like Kafka clusters for application performance testing and DR testing scenarios. A production environment is not usually a single Kafka cluster either, it can be multiple clusters optimized for different purposes. You may want a self-managed Kafka cluster to deploy on your edge clusters that run offline, on-premise infrastructure that may require a non-standard topology or public cloud with a fairly standard multi-AZ deployment. And there are many self-managed Kafka platforms from Red Hat, Confluent, Cloudera, TIBCO, to name a few. The main characteristic of a self-managed cluster is the fact that the responsibility to manage and run the Kafka cluster resides within the organization owning the cluster. With that, a self-managed cluster also allows customization and configuration of the Kafka cluster, bespoke tuning to suit your deployment needs. For these and any other odd use cases that are not possible with Kafka as a Service model, the self-managed Kafka remains a proven path.
Kafka as a Service
Each organization is different, but some of the common criteria for production Kafka clusters are things such as the ability to deploy on multiple AZs, on-demand scaling, compliance and certifications, a predictable cost model, open for 3rd party tools and services integrations, and so forth. Today, Kafka is over a decade old and there are multiple mature Kafka as a Service offerings able to satisfy many production needs. While these offerings vary in sizing options, the richness of the user interface, Kafka ecosystem components, and so forth, a key difference is whether Kafka is treated as an infrastructure component or treated as its own event-streaming category with its event-streaming abstractions.
Apache Kafka distributions landscape
Based on the abstraction criteria we can see that some SaaS providers (such as AWS MSK, Heroku, Instaclustr, Aiven) focus on infrastructure details such as VM sizes, the number of cores and memory, storage types, broker, Zookeeper, Kafka Connect details, and so forth. Many critical decisions about the infra choice, capacity matching to Kafka, Kafka cluster configurations, Zookeeper topology, are left for the user to decide. These services resemble infrastructure services that happen to run Kafka on top, and that is reflected in the VM-based sizing and pricing models. These services have a larger selection of sizing options and can be preferred by teams that have infrastructure inclination and preference to know and control all aspects of a service (even a managed service).
Other Kafka as a Service providers (such as Confluent Cloud, AWS MSK Serverless, Red Hat Openshift Streams for Apache Kafka, Upstash) go the opposite “Kafka-first” direction where the infra, the management layer (typically Kubernetes based), and Kafka cluster details are taken care of, and hidden. With these services, the user is dealing with higher level, Kafka-focused abstractions such as Streaming/Kafka/Topic-like units of measure (which represents normalized multi-dimensional Kafka capacity) rather than infrastructure capacity; availability guarantees instead of deployment topology of brokers and Zookeeper; connectors to external systems as an API (regardless of the implementation technology) instead of Kafka Connect cluster deployment and connector deployments. This approach exposes what is important for a Kafka user and not the underlying infrastructure or implementation choices that make up a Kafka service. In addition, these Kafka-first services offer a consumption based Kafka-centric pricing model where the user pays for Kafka capacity used and quality of service rather than provisioned infrastructure with the additional Kafka margin. These services are more suitable for lines of business teams that focus on their business domain and treat Kafka as a commodity tool to solve the business challenges.
Next, we will see why Kafka-first managed services are blurring the line and getting closer to a serverless-like Kafka experience where the user is interacting with Kafka APIs and everything else is taken care of.
“Serverless-like” Kafka
Serverless technologies are a class of SaaS that have specific characteristics offering additional benefits to users such as a pay-per-use pricing model and eliminating the need for capacity management and scaling altogether. This is achieved through things such as not having to provision and manage servers, built-in high availability, built-in rebalancing, automatic scaling up, and scaling down to zero.
We can look at the “Serverless Kafka” category from two opposing angles. On the positive side, we can say that the “Kafka-first” services are getting pretty close to a serverless user experience except for the pricing aspect. With these Kafka-first services, users don't have to provision infrastructure, the Kafka clusters are already preconfigured for high availability, with partition rebalancing, storage expansion, and auto-scaling (within certain boundaries).
On the negative side, whether a Kafka service is called serverless or not, these offerings still have significant technical and pricing limitations and they are not mature enough. These services are constrained in terms of message size, partition count, partition limit, network limit, storage limit. These constraints limit the use cases where a so-called serverless Kafka can be used. Other than Upstash which is charging per message, the remaining serverless Kafka services charge for cluster hours which is against the scale-to-zero/pay-per-use ethos of the serverless definition.
That is why today I consider the serverless Kafka category still an inspiration rather than reality. Nevertheless, these trends set the direction where managed Kafka offerings are headed: that is complete infrastructure and deployment abstractions hidden from the user; Kafka-first primitives for capacity, usage, quality of a service; autonomous service lifecycle that doesn’t require any user intervention; and with a true pay-for-what-you-use pricing model.
Summary
How many types of Kafka do you need? The answer is more than one. You want developer frameworks that can emulate Kafka locally and enable rapid, iterative development. You want a declarative and automated way to repeatedly deploy and update development environments. Depending on your business requirements, you may require highly customised Kafka implementations at the edge or standard implementations across multiple clouds that are all connected. While your organization's event streaming adoption and Kafka maturity grows, you will need more Kafka. But there is a paradox. If you are not in the Kafka business, you should work less on Kafka itself and use Kafka for more tasks that set your business apart. This is possible if you use Kafka through higher-level frameworks like Strimzi that automate many of the operational aspects, or through a Kafka-first service that takes care of low-level decision-making and relieves you of the responsibility of running Kafka. This way, your teams stop thinking about Kafka and start thinking about how to use Kafka for what matters to your customers.
Follow me at @bibryam to join my journey of learning Apache Kafka. This post was originally published on Red Hat Developers. To read the original post, check here and here.
Kafka Optimization Theorem
One of the foundational theorems in distributed systems is the CAP theorem. It simply explains why a distributed data store can provide only two (CP or AP, since P is a given) of the consistency (C), availability(A), and partition tolerance(P) guarantees. While this dilemma explains the main tradeoffs of distributed systems in the case of network partitions, it is not useful in the day-to-day optimizing of systems for specific performance goals in the happy path scenarios. In addition to consistency and availability, developers have to optimize applications for latency and throughput too. In this post, we will uncover how the main distributed systems forces relate to each other and which are the main primitives influencing these forces in the context of Apache Kafka. We will visualize and formulate these findings in the form of a theorem that can serve as a guide while optimizing Apache Kafka for specific goals.
Kafka Primitives
One of the less-known distributed systems theorems is PACELC which extends on CAP by explaining what else (E) happens when a distributed system is running normally in the absence of partitioning. In that case, one can optimize for latency (L) or consistency (C) (hence the full acronym PAC-E-LC). PACELC explains how some systems such as Amazon’s Dynamo favor availability and lower latency over consistency, and how ACID-compliant systems favor consistency over availability or lower latency.
PACELC theorem for distributed data stores
While PACELC explains the main tradeoffs and distributed data stores, it doesn’t apply as-is to Kafka. Kafka is an event streaming platform used primarily for moving data from one place to another. A better way to look at a Kafka-based system is as a collection of producers, consumers, and topics forming distinct data flows. The consequence of that is, the client application configuration can influence every goal significantly and there are first class optimization goals in streaming applications such as end-to-end latency and throughput. Whether you are aware of it or not, every time you configure a Kafka cluster, create a topic, or configure a producer or a consumer, you are making a choice between latency vs throughput and availability vs durability. To explain why this is the case, we have to look into the main dimensions of Kafka topics and the role client applications play in an event flow.
Apache Kafka optimization goals
Partitions
The topic partitions (on the X-axis in our diagram) represent the unit of parallelism in Kafka. On the broker, and the client-side, reads and writes to different partitions can be done fully in parallel. While there are many factors that can limit throughput, generally a higher number of partitions for a topic will enable higher throughput, and a smaller number of partitions will lead to lower throughput.
At the same time, a very large number of partitions will lead to the creation of more metadata that needs to be passed and processed across all brokers and clients. This can impact end to end latency negatively unless more resources are added to the brokers. This is a purposely simplified view of partitions to demonstrate the main tradeoff that the number of partitions leads to in our context.
Replicas
The replication factor (on the Y-axis in our diagram) determines the number of copies (including the leader) each topic partition in a cluster must have. By ensuring all replicas of a partition exist on different brokers, replicas define the data durability. A higher number of replicas ensure the data is copied to more brokers and ensure better durability of data in the case of broker failure.
On the other hand, a lower number of replicas reduces the data durability but in certain circumstances it can increase the availability for the producers or consumers by tolerating more broker failures. That said, the availability for a consumer is determined by the availability of in-sync replicas, whereas for a producer it is determined by the minimum number of in-sync replicas. With a low number of replicas, the availability of overall data flow will be dependent on which brokers fail (the one with the partitions leader of interest or not) and if other brokers are in sync or not. For our purpose we assume less replicas would lead to higher application availability and lower data durability.
Partitions and replicas are not the only primitives influencing the tradeoff between throughput vs latency and durability vs availability, but they represent the broker side of the picture. Other participants in shaping the main Kafka optimization tradeoffs are the client applications.
Producers and Consumers
Topics are used by the producers that send messages and consumers that read these messages. Producers and consumers also state their preference between throughput vs latency and durability vs availability through various configuration options. It is the combination of topic and client application configurations (and other cluster-level configurations such as leader election) that defines what your application is optimized for.
We can look at a flow of events consisting of a producer, a consumer, and a topic. Optimizing such an event flow for average latency, on the client-side would require tuning the producer and consumer to exchange smaller batches of messages. The same flow can be tuned for average throughput by configuring the producer and consumer for larger batches of messages. In the same way, the number of topic partitions influences throughput vs latency, a producer and consumer message batch sizes influence the same.
Producers and consumers involved in a message flow state preference for durability or availability too. A producer that favors durability over availability can demand a higher number of acknowledgements by specifying acks=all. A lower number of acknowledgments (lower than minISR which means 0 or 1) could lead to higher availability from the producer point of view by tolerating a higher number of broker failures, but reduces data durability in the case of catastrophic events with the brokers.
The consumer configurations influencing this dimension (Y-axis) are not as straightforward as the producer configurations but dependent on the consumer application logic. A consumer can favor higher consistency by committing message consumption offsets more often or even individually. Or the consumer can favor availability by increasing various timeouts and tolerating broker failures for longer.
Kafka Optimization Theorem
We defined the main actors involved in an event flow as a producer, a consumer, and a topic. We also defined the opposing goals we have to optimize for: throughput vs latency and durability vs availability. Given that, the Kafka Optimization Theorem states that any Kafka data flow makes tradeoffs between throughput vs latency and durability vs availability.
Kafka Optimization Theorem with primary configuration options
For simplicity, we put producer and consumer preferences on the same axes as topic replicas and partitions. Optimizing for a particular goal would be easier to achieve when these primitives are aligned. For example, optimizing an end-to-end data flow for low latency would be best achieved with smaller producer and consumer message batches combined with a small number of partitions.
A data flow optimized for higher throughput would have larger producer and consumer message batches and higher number of partitions for parallel processing. A data flow optimized for durability would have a higher number of replicas and require a higher number of producer acknowledgments and granular consumer commits. If the data flow is optimized for availability, a smaller number of replicas, and/or smaller number of producer acknowledgments, larger timeouts, would be preferred. In practice, there is no correlation between partitions, replicas, producers, and consumers configurations. It is possible to have a large number of replicas for a topic (minISR), but have a producer that requires a 0 or 1 of acknowledgment. Or have a higher number of partitions because your application logic requires so, and small producer and consumer message batches. These are valid scenarios to use Kafka and one of the strengths of Apache Kafka is being a highly configurable and flexible eventing system satisfying many use cases. The framework proposed here is to serve as a mental model for the main Kafka primitives and how they relate to the optimization dimensions. Knowing the foundational forces will enable you to do tuning specific for your application and understand the effects.
The Golden Ratio
In the proposed Kafka Optimization Theorem, there are deliberately no numbers but only the relation between the main primitives and the direction of change. It is not intended to serve as a concrete optimization configuration but a guide that shows how reducing or increasing a primitive configuration influences the direction of the optimization. Yet sharing a few of the most common configuration options accepted as the industry best practices could be useful as a starting point and demonstration of the theorem in practice.
Most Kafka clusters today, whether that is on-prem with something like Strimzi, or as a fully managed service offering such as OpenShift Streams for Apache Kafka, are almost always deployed within a single region. A production-grade Kafka cluster is typically spread into 3 availability zones (AZs) with a replication factor of 3 (RF=3) and minimum in-sync replicas of 2 (minISR=2). This ensures a good level of data durability during happy times, and good availability for client applications during temporary disruptions. This represents the good middle ground as having minISR=3 would prevent producers from producing even when a single broker is affected, and having minISR=1 would affect both producer and consumer when a leader is down. Typically this replication configuration is accompanied by acks=all on the producer side and default offset commit configurations for the consumers.
While there are commonalities in consistency and availability tradeoffs among different applications, throughput and latency requirements vary. The number of partitions for a topic is influenced by the shape of the data, the data processing logic, and its ordering requirements. At the same time, the number of partitions dictates what is the level of maximum parallelism and message throughput you can achieve. As a consequence, there is no good default number or a range for the partition count.
By default, Kafka clients are optimized for low latency. We can observe that from the default producer values (batch.size=16384, linger.ms=0, compression.type=none) and the default consumer values (fetch.min.bytes=1, fetch.max.wait.ms=500). The producer prior Kafka 3 had acks=1 which recently changed to acks=all with a preference on durability rather than availability or low latency. Optimizing the client applications for throughput would require increasing wait times and batch sizes 5-10 fold and examining the results. Knowing the default values and what they are optimized for is a good starting point for your service optimization goal.
Summary
CAP is a great theorem for failure scenarios. While failures are a given and partitioning will always happen, we have to optimize applications for happy paths too. This post introduces a simplified model explaining how Kafka primitives can be used for performance optimization. It deliberately focuses on the main primitives and selective configurations options to demonstrate the principles. In real life, more factors influence your application performance metrics. Encryption, compression, and memory allocation will impact latency and throughput. Transactions, exactly-one semantics, retries, message flushes, and leader election will impact consistency and availability tradeoffs. You will have broker primitives (replicas and partitions) and client primitives (batch sizes and acknowledgments) optimized for competing tradeoffs. Finding the ideal Kafka configuration for your application will require experimentation, and the Kafka Optimization Theorem will guide you in the journey. Follow me at @bibryam to join my journey of learning Apache Kafka. This post was originally published on Red Hat Developers. To read the original post, check here.